Plotting top tree
loss counties
# Summary statistics
# Merging projected SF to GFW data frame
wus_loss_merge <- wus_counties_sf_pro %>%
left_join(Wustclsubset, by = c("STATE_NAME" = "subnational1", "NAME" = "subnational2"))
# Replacing NA's with zero
total_loss_by_county <- wus_loss_merge %>%
mutate(across(starts_with("tc_loss_ha_"), as.numeric, .names = "numeric_{.col}")) %>%
rowwise() %>%
mutate(loss_2000_2020_ha = sum(c_across(starts_with("numeric_tc_loss_ha_")), na.rm = TRUE)) %>%
ungroup() %>%
select(-starts_with("numeric_tc_loss_ha_")) # Removing temporary numeric columns
head(wus_loss_merge)
## Simple feature collection with 6 features and 40 fields
## Geometry type: MULTIPOLYGON
## Dimension: XY
## Bounding box: xmin: -1833052 ymin: 504019.9 xmax: -640734.2 ymax: 2117980
## Projected CRS: +proj=laea +lat_0=30 +lon_0=-100
## STATEFP COUNTYFP COUNTYNS AFFGEOID GEOID NAME NAMELSAD STUSPS STATE_NAME LSAD
## 1 30 009 01720111 0500000US30009 30009 Carbon Carbon County MT Montana 06
## 2 16 007 00395090 0500000US16007 16007 Bear Lake Bear Lake County ID Idaho 06
## 3 41 035 01155134 0500000US41035 41035 Klamath Klamath County OR Oregon 06
## 4 53 043 01514052 0500000US53043 53043 Lincoln Lincoln County WA Washington 06
## 5 06 059 00277294 0500000US06059 06059 Orange Orange County CA California 06
## 6 06 111 00277320 0500000US06111 06111 Ventura Ventura County CA California 06
## ALAND AWATER country threshold area_ha extent_2000_ha extent_2010_ha gain_2000_2020_ha
## 1 5303728455 35213028 United States 50 533358 53663 36426 385
## 2 2527123155 191364281 United States 50 271541 44456 31959 486
## 3 15410373389 484953082 United States 50 1590318 487365 427271 72164
## 4 5984421204 75265950 United States 50 605748 8713 12163 961
## 5 2053476505 406279630 United States 50 206072 10626 14559 3478
## 6 4767622161 947345735 United States 50 480834 67109 76605 2626
## tc_loss_ha_2001 tc_loss_ha_2002 tc_loss_ha_2003 tc_loss_ha_2004 tc_loss_ha_2005 tc_loss_ha_2006
## 1 235 695 92 237 326 61
## 2 22 8 1 93 34 9
## 3 4601 4274 6348 5393 3755 2991
## 4 68 98 5 378 38 180
## 5 75 108 66 84 69 481
## 6 144 1941 1957 1267 849 10156
## tc_loss_ha_2007 tc_loss_ha_2008 tc_loss_ha_2009 tc_loss_ha_2010 tc_loss_ha_2011 tc_loss_ha_2012
## 1 887 880 310 20 644 203
## 2 11 4 9 40 164 138
## 3 3675 3548 4954 1767 2594 2315
## 4 92 30 75 19 8 25
## 5 784 305 101 25 11 60
## 6 3174 1348 105 118 61 100
## tc_loss_ha_2013 tc_loss_ha_2014 tc_loss_ha_2015 tc_loss_ha_2016 tc_loss_ha_2017 tc_loss_ha_2018
## 1 41 188 135 5 1 89
## 2 58 50 2 13 68 1
## 3 2456 3880 4547 5318 8485 7872
## 4 21 33 46 93 108 74
## 5 0 4 1 19 24 854
## 6 487 81 47 207 14992 2947
## tc_loss_ha_2019 tc_loss_ha_2020 tc_loss_ha_2021 tc_loss_ha_2022 geometry
## 1 70 0 2758 255 MULTIPOLYGON (((-775324.9 1...
## 2 137 2 1 3 MULTIPOLYGON (((-957856.1 1...
## 3 5236 4434 27950 4287 MULTIPOLYGON (((-1829815 15...
## 4 72 37 36 54 MULTIPOLYGON (((-1436119 20...
## 5 357 17 14 71 MULTIPOLYGON (((-1666632 55...
## 6 263 36 19 29 MULTIPOLYGON (((-1781105 60...
head(total_loss_by_county)
## Simple feature collection with 6 features and 41 fields
## Geometry type: MULTIPOLYGON
## Dimension: XY
## Bounding box: xmin: -1833052 ymin: 504019.9 xmax: -640734.2 ymax: 2117980
## Projected CRS: +proj=laea +lat_0=30 +lon_0=-100
## # A tibble: 6 × 42
## STATEFP COUNTYFP COUNTYNS AFFGEOID GEOID NAME NAMELSAD STUSPS STATE_NAME LSAD ALAND AWATER country
## <chr> <chr> <chr> <chr> <chr> <chr> <chr> <chr> <chr> <chr> <dbl> <dbl> <chr>
## 1 30 009 01720111 0500000U… 30009 Carb… Carbon … MT Montana 06 5.30e 9 3.52e7 United…
## 2 16 007 00395090 0500000U… 16007 Bear… Bear La… ID Idaho 06 2.53e 9 1.91e8 United…
## 3 41 035 01155134 0500000U… 41035 Klam… Klamath… OR Oregon 06 1.54e10 4.85e8 United…
## 4 53 043 01514052 0500000U… 53043 Linc… Lincoln… WA Washington 06 5.98e 9 7.53e7 United…
## 5 06 059 00277294 0500000U… 06059 Oran… Orange … CA California 06 2.05e 9 4.06e8 United…
## 6 06 111 00277320 0500000U… 06111 Vent… Ventura… CA California 06 4.77e 9 9.47e8 United…
## # ℹ 29 more variables: threshold <dbl>, area_ha <dbl>, extent_2000_ha <dbl>, extent_2010_ha <dbl>,
## # gain_2000_2020_ha <dbl>, tc_loss_ha_2001 <dbl>, tc_loss_ha_2002 <dbl>, tc_loss_ha_2003 <dbl>,
## # tc_loss_ha_2004 <dbl>, tc_loss_ha_2005 <dbl>, tc_loss_ha_2006 <dbl>, tc_loss_ha_2007 <dbl>,
## # tc_loss_ha_2008 <dbl>, tc_loss_ha_2009 <dbl>, tc_loss_ha_2010 <dbl>, tc_loss_ha_2011 <dbl>,
## # tc_loss_ha_2012 <dbl>, tc_loss_ha_2013 <dbl>, tc_loss_ha_2014 <dbl>, tc_loss_ha_2015 <dbl>,
## # tc_loss_ha_2016 <dbl>, tc_loss_ha_2017 <dbl>, tc_loss_ha_2018 <dbl>, tc_loss_ha_2019 <dbl>,
## # tc_loss_ha_2020 <dbl>, tc_loss_ha_2021 <dbl>, tc_loss_ha_2022 <dbl>, geometry <MULTIPOLYGON [m]>, …
summary(total_loss_by_county$loss_2000_2020_ha)
## Min. 1st Qu. Median Mean 3rd Qu. Max.
## 0 1380 12800 36004 49959 335182
# Histogram
ggplot(total_loss_by_county, aes(x = loss_2000_2020_ha)) +
geom_histogram(binwidth = 200, fill = "skyblue", color = "black") +
labs(title = "Histogram", x = "Tree Cover Gain (ha)", y = "Frequency")
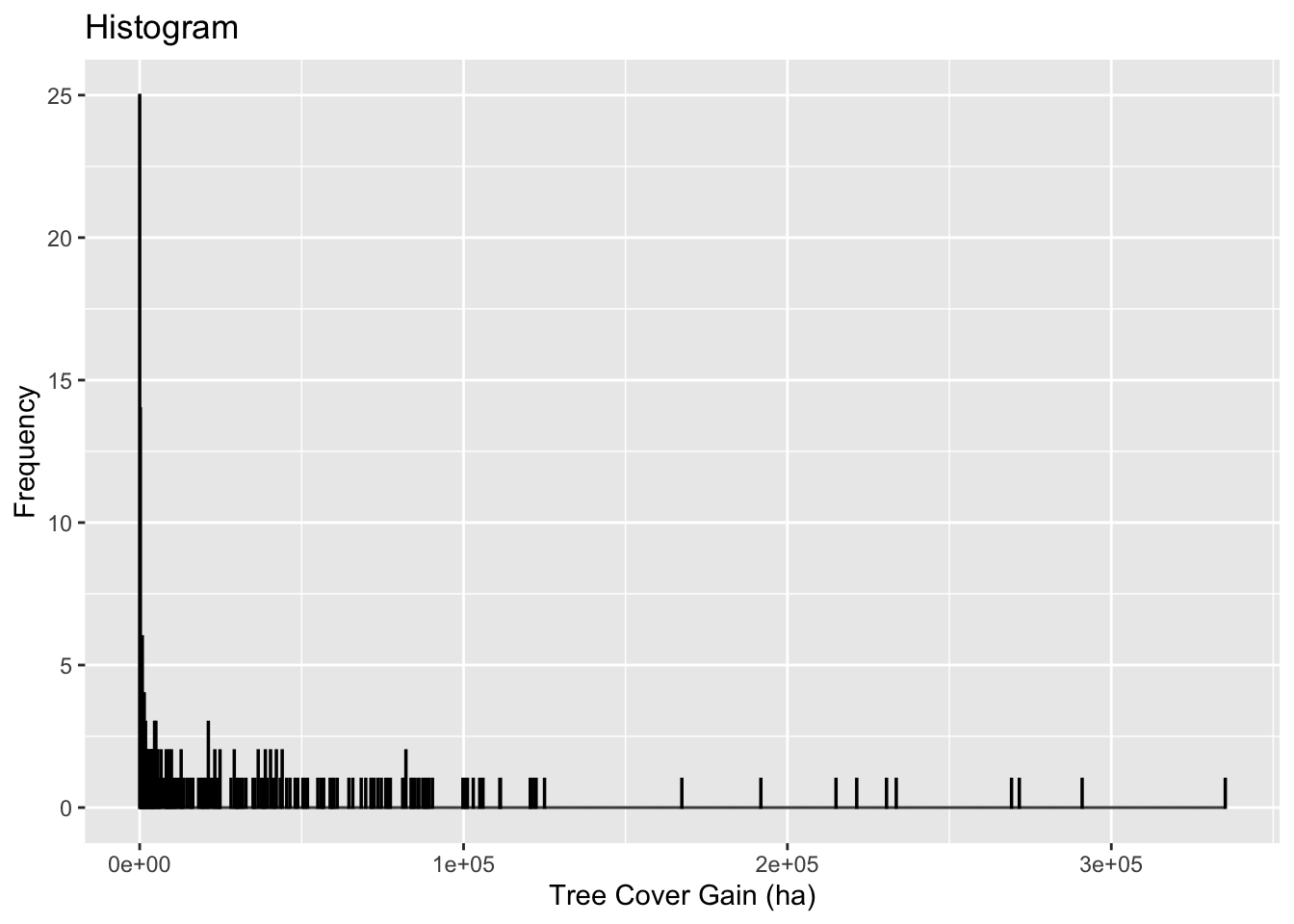
# Boxplot highlighting PNW outliers by state
PNW <- c("Washington", "Oregon", "California", "Idaho", "Montana")
PNW_SF <- total_loss_by_county[total_loss_by_county$STATE_NAME %in% PNW, ]
head(PNW_SF)
## Simple feature collection with 6 features and 41 fields
## Geometry type: MULTIPOLYGON
## Dimension: XY
## Bounding box: xmin: -1833052 ymin: 504019.9 xmax: -640734.2 ymax: 2117980
## Projected CRS: +proj=laea +lat_0=30 +lon_0=-100
## # A tibble: 6 × 42
## STATEFP COUNTYFP COUNTYNS AFFGEOID GEOID NAME NAMELSAD STUSPS STATE_NAME LSAD ALAND AWATER country
## <chr> <chr> <chr> <chr> <chr> <chr> <chr> <chr> <chr> <chr> <dbl> <dbl> <chr>
## 1 30 009 01720111 0500000U… 30009 Carb… Carbon … MT Montana 06 5.30e 9 3.52e7 United…
## 2 16 007 00395090 0500000U… 16007 Bear… Bear La… ID Idaho 06 2.53e 9 1.91e8 United…
## 3 41 035 01155134 0500000U… 41035 Klam… Klamath… OR Oregon 06 1.54e10 4.85e8 United…
## 4 53 043 01514052 0500000U… 53043 Linc… Lincoln… WA Washington 06 5.98e 9 7.53e7 United…
## 5 06 059 00277294 0500000U… 06059 Oran… Orange … CA California 06 2.05e 9 4.06e8 United…
## 6 06 111 00277320 0500000U… 06111 Vent… Ventura… CA California 06 4.77e 9 9.47e8 United…
## # ℹ 29 more variables: threshold <dbl>, area_ha <dbl>, extent_2000_ha <dbl>, extent_2010_ha <dbl>,
## # gain_2000_2020_ha <dbl>, tc_loss_ha_2001 <dbl>, tc_loss_ha_2002 <dbl>, tc_loss_ha_2003 <dbl>,
## # tc_loss_ha_2004 <dbl>, tc_loss_ha_2005 <dbl>, tc_loss_ha_2006 <dbl>, tc_loss_ha_2007 <dbl>,
## # tc_loss_ha_2008 <dbl>, tc_loss_ha_2009 <dbl>, tc_loss_ha_2010 <dbl>, tc_loss_ha_2011 <dbl>,
## # tc_loss_ha_2012 <dbl>, tc_loss_ha_2013 <dbl>, tc_loss_ha_2014 <dbl>, tc_loss_ha_2015 <dbl>,
## # tc_loss_ha_2016 <dbl>, tc_loss_ha_2017 <dbl>, tc_loss_ha_2018 <dbl>, tc_loss_ha_2019 <dbl>,
## # tc_loss_ha_2020 <dbl>, tc_loss_ha_2021 <dbl>, tc_loss_ha_2022 <dbl>, geometry <MULTIPOLYGON [m]>, …
quantile(total_loss_by_county$loss_2000_2020_ha)
## 0% 25% 50% 75% 100%
## 0.00 1379.75 12800.50 49959.25 335182.00
ggplot(PNW_SF, aes(x = STATE_NAME, y = loss_2000_2020_ha)) +
geom_boxplot(width = 0.5, outlier.shape = 8, outlier.color = "red", outlier.size = 3) +
labs(title = "Boxplot of Tree Cover Gain by State", y = "Tree Cover Gain (ha)", x = "") +
facet_wrap( ~ STATE_NAME, scales = "free_x", ncol = 2) +
theme_minimal() +
theme(plot.title = element_text(face = "bold"),
legend.position = "none",
axis.text.x = element_blank(),
axis.ticks.x = element_blank()) +
coord_cartesian(ylim = c(-0, 100000))
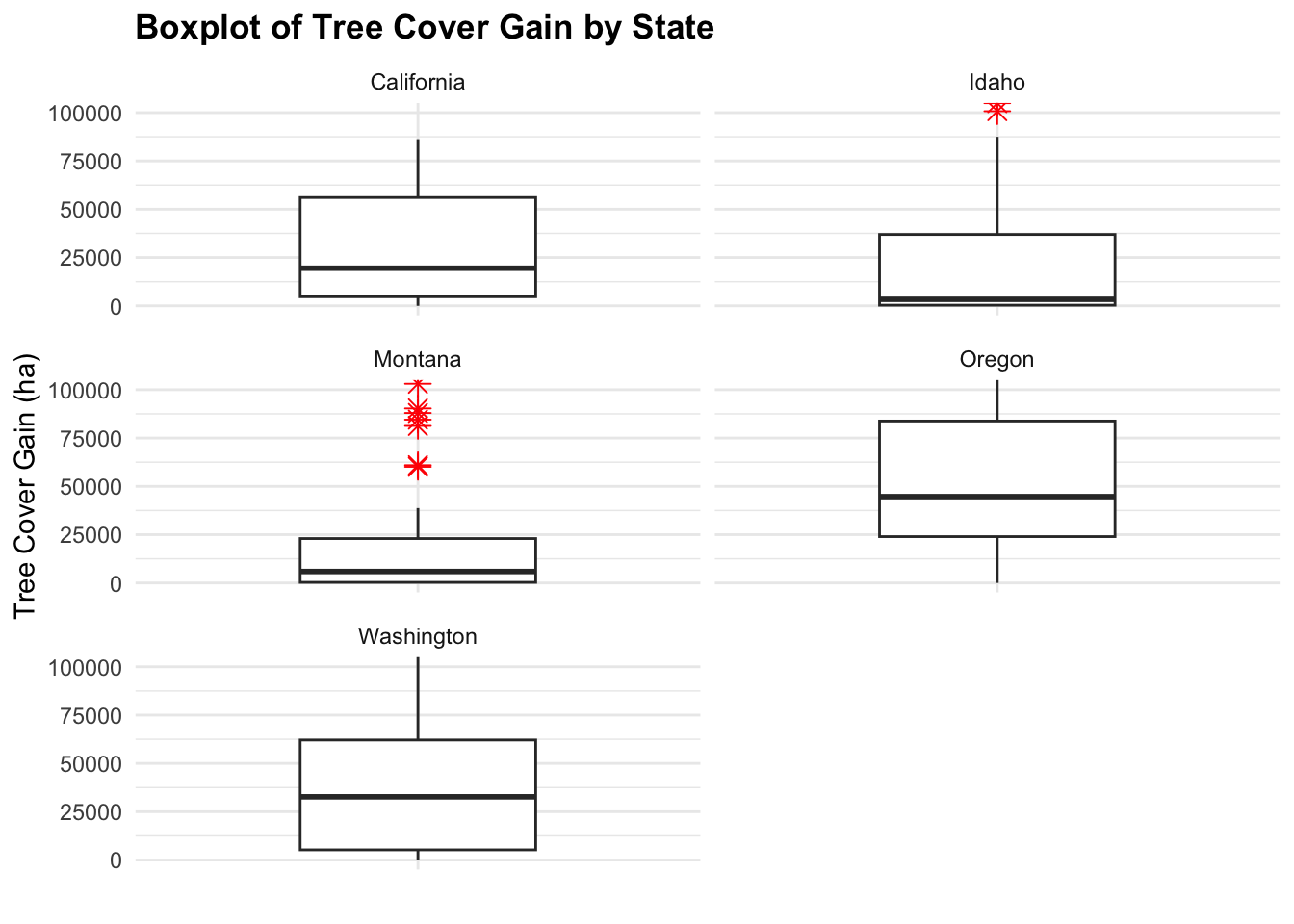
Visualizing on a
plot
# Merging projected SF to GFW data frame
wus_loss_merge <- wus_counties_sf_pro %>%
left_join(Wustclsubset, by = c("STATE_NAME" = "subnational1", "NAME" = "subnational2"))
# Replacing NA's with zero
total_loss_by_county <- wus_loss_merge %>%
mutate(across(starts_with("tc_loss_ha_"), as.numeric, .names = "numeric_{.col}")) %>%
rowwise() %>%
mutate(loss_2000_2020_ha = sum(c_across(starts_with("numeric_tc_loss_ha_")), na.rm = TRUE)) %>%
ungroup() %>%
select(-starts_with("numeric_tc_loss_ha_")) # Removing temporary numeric columns
head(wus_loss_merge)
## Simple feature collection with 6 features and 40 fields
## Geometry type: MULTIPOLYGON
## Dimension: XY
## Bounding box: xmin: -1833052 ymin: 504019.9 xmax: -640734.2 ymax: 2117980
## Projected CRS: +proj=laea +lat_0=30 +lon_0=-100
## STATEFP COUNTYFP COUNTYNS AFFGEOID GEOID NAME NAMELSAD STUSPS STATE_NAME LSAD
## 1 30 009 01720111 0500000US30009 30009 Carbon Carbon County MT Montana 06
## 2 16 007 00395090 0500000US16007 16007 Bear Lake Bear Lake County ID Idaho 06
## 3 41 035 01155134 0500000US41035 41035 Klamath Klamath County OR Oregon 06
## 4 53 043 01514052 0500000US53043 53043 Lincoln Lincoln County WA Washington 06
## 5 06 059 00277294 0500000US06059 06059 Orange Orange County CA California 06
## 6 06 111 00277320 0500000US06111 06111 Ventura Ventura County CA California 06
## ALAND AWATER country threshold area_ha extent_2000_ha extent_2010_ha gain_2000_2020_ha
## 1 5303728455 35213028 United States 50 533358 53663 36426 385
## 2 2527123155 191364281 United States 50 271541 44456 31959 486
## 3 15410373389 484953082 United States 50 1590318 487365 427271 72164
## 4 5984421204 75265950 United States 50 605748 8713 12163 961
## 5 2053476505 406279630 United States 50 206072 10626 14559 3478
## 6 4767622161 947345735 United States 50 480834 67109 76605 2626
## tc_loss_ha_2001 tc_loss_ha_2002 tc_loss_ha_2003 tc_loss_ha_2004 tc_loss_ha_2005 tc_loss_ha_2006
## 1 235 695 92 237 326 61
## 2 22 8 1 93 34 9
## 3 4601 4274 6348 5393 3755 2991
## 4 68 98 5 378 38 180
## 5 75 108 66 84 69 481
## 6 144 1941 1957 1267 849 10156
## tc_loss_ha_2007 tc_loss_ha_2008 tc_loss_ha_2009 tc_loss_ha_2010 tc_loss_ha_2011 tc_loss_ha_2012
## 1 887 880 310 20 644 203
## 2 11 4 9 40 164 138
## 3 3675 3548 4954 1767 2594 2315
## 4 92 30 75 19 8 25
## 5 784 305 101 25 11 60
## 6 3174 1348 105 118 61 100
## tc_loss_ha_2013 tc_loss_ha_2014 tc_loss_ha_2015 tc_loss_ha_2016 tc_loss_ha_2017 tc_loss_ha_2018
## 1 41 188 135 5 1 89
## 2 58 50 2 13 68 1
## 3 2456 3880 4547 5318 8485 7872
## 4 21 33 46 93 108 74
## 5 0 4 1 19 24 854
## 6 487 81 47 207 14992 2947
## tc_loss_ha_2019 tc_loss_ha_2020 tc_loss_ha_2021 tc_loss_ha_2022 geometry
## 1 70 0 2758 255 MULTIPOLYGON (((-775324.9 1...
## 2 137 2 1 3 MULTIPOLYGON (((-957856.1 1...
## 3 5236 4434 27950 4287 MULTIPOLYGON (((-1829815 15...
## 4 72 37 36 54 MULTIPOLYGON (((-1436119 20...
## 5 357 17 14 71 MULTIPOLYGON (((-1666632 55...
## 6 263 36 19 29 MULTIPOLYGON (((-1781105 60...
head(total_loss_by_county)
## Simple feature collection with 6 features and 41 fields
## Geometry type: MULTIPOLYGON
## Dimension: XY
## Bounding box: xmin: -1833052 ymin: 504019.9 xmax: -640734.2 ymax: 2117980
## Projected CRS: +proj=laea +lat_0=30 +lon_0=-100
## # A tibble: 6 × 42
## STATEFP COUNTYFP COUNTYNS AFFGEOID GEOID NAME NAMELSAD STUSPS STATE_NAME LSAD ALAND AWATER country
## <chr> <chr> <chr> <chr> <chr> <chr> <chr> <chr> <chr> <chr> <dbl> <dbl> <chr>
## 1 30 009 01720111 0500000U… 30009 Carb… Carbon … MT Montana 06 5.30e 9 3.52e7 United…
## 2 16 007 00395090 0500000U… 16007 Bear… Bear La… ID Idaho 06 2.53e 9 1.91e8 United…
## 3 41 035 01155134 0500000U… 41035 Klam… Klamath… OR Oregon 06 1.54e10 4.85e8 United…
## 4 53 043 01514052 0500000U… 53043 Linc… Lincoln… WA Washington 06 5.98e 9 7.53e7 United…
## 5 06 059 00277294 0500000U… 06059 Oran… Orange … CA California 06 2.05e 9 4.06e8 United…
## 6 06 111 00277320 0500000U… 06111 Vent… Ventura… CA California 06 4.77e 9 9.47e8 United…
## # ℹ 29 more variables: threshold <dbl>, area_ha <dbl>, extent_2000_ha <dbl>, extent_2010_ha <dbl>,
## # gain_2000_2020_ha <dbl>, tc_loss_ha_2001 <dbl>, tc_loss_ha_2002 <dbl>, tc_loss_ha_2003 <dbl>,
## # tc_loss_ha_2004 <dbl>, tc_loss_ha_2005 <dbl>, tc_loss_ha_2006 <dbl>, tc_loss_ha_2007 <dbl>,
## # tc_loss_ha_2008 <dbl>, tc_loss_ha_2009 <dbl>, tc_loss_ha_2010 <dbl>, tc_loss_ha_2011 <dbl>,
## # tc_loss_ha_2012 <dbl>, tc_loss_ha_2013 <dbl>, tc_loss_ha_2014 <dbl>, tc_loss_ha_2015 <dbl>,
## # tc_loss_ha_2016 <dbl>, tc_loss_ha_2017 <dbl>, tc_loss_ha_2018 <dbl>, tc_loss_ha_2019 <dbl>,
## # tc_loss_ha_2020 <dbl>, tc_loss_ha_2021 <dbl>, tc_loss_ha_2022 <dbl>, geometry <MULTIPOLYGON [m]>, …
# Classifying data for visualization using natural jenks method
ctpnts <- classIntervals(total_loss_by_county$loss_2000_2020_ha, n = 6, style = "jenks")
cols <- brewer.pal(6, "YlOrRd")
colors <- colorRampPalette(cols)(length(ctpnts$brks)-1)
# Plotting
ggplot(total_loss_by_county) +
geom_sf(aes(fill = loss_2000_2020_ha)) +
scale_fill_gradientn(colors = colors,
breaks = ctpnts$brks[-length(ctpnts$brks)],
labels = rev(paste(round(ctpnts$brks[-length(ctpnts$brks)], 1), "to",
round(ctpnts$brks[-1], 1))),
limits = range(total_loss_by_county$loss_2000_2020_ha)) +
labs(title = "20 Year Forest Cover Loss (50% threshold)", fill = "Loss (hectares)") +
theme(plot.title = element_text(hjust = 0.5, face = "bold"),
legend.position = "right",
legend.background = element_rect(fill = "white", colour = "black"),
legend.key = element_blank(),
legend.text = element_text(size = 12),
legend.title = element_text(size = 14)) +
guides(fill = guide_legend(override.aes = list(fill = rev(colors)))) +
geom_sf(data = wus_states_sf_pro, fill = NA, color = "black", size = 1.5)
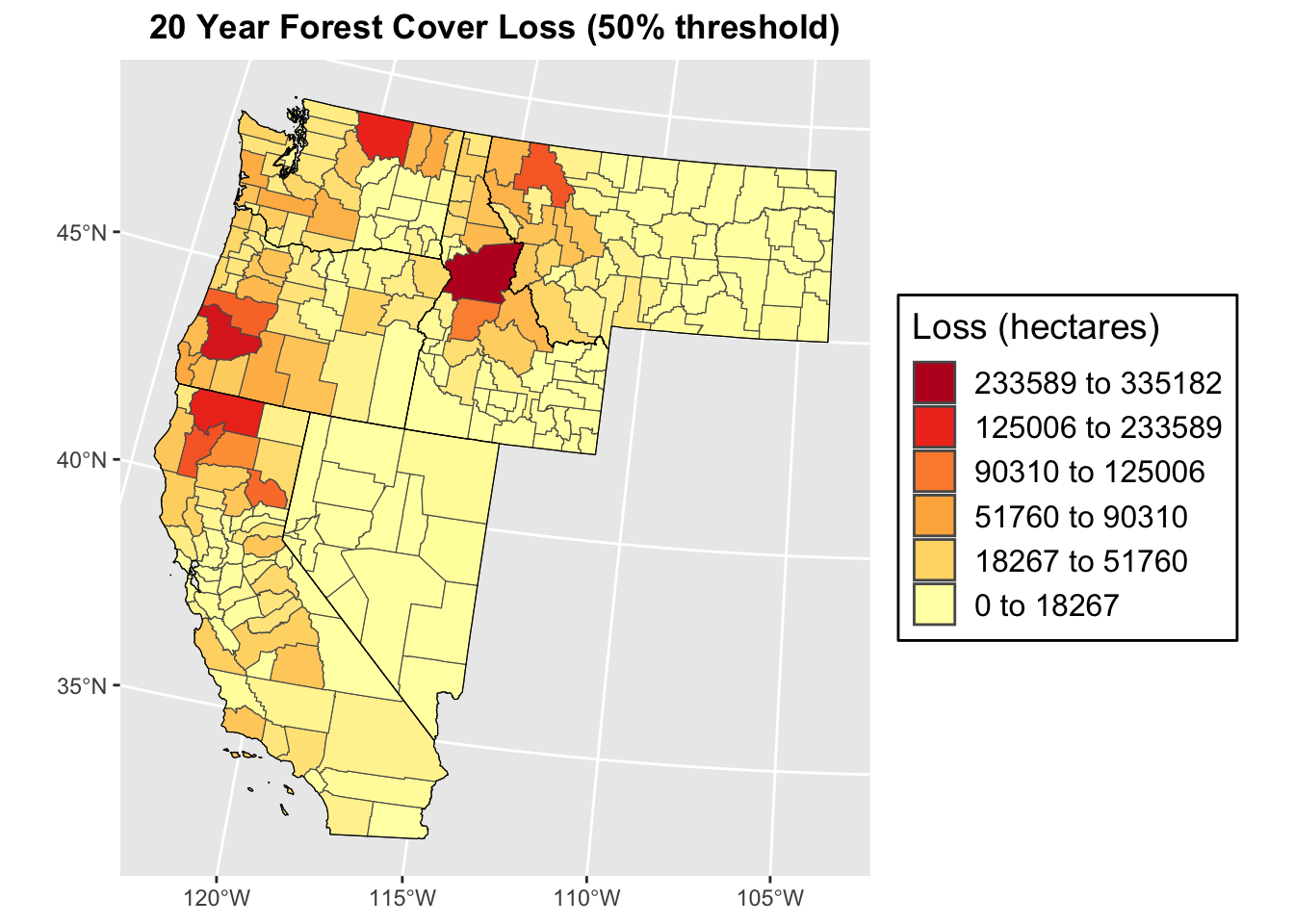